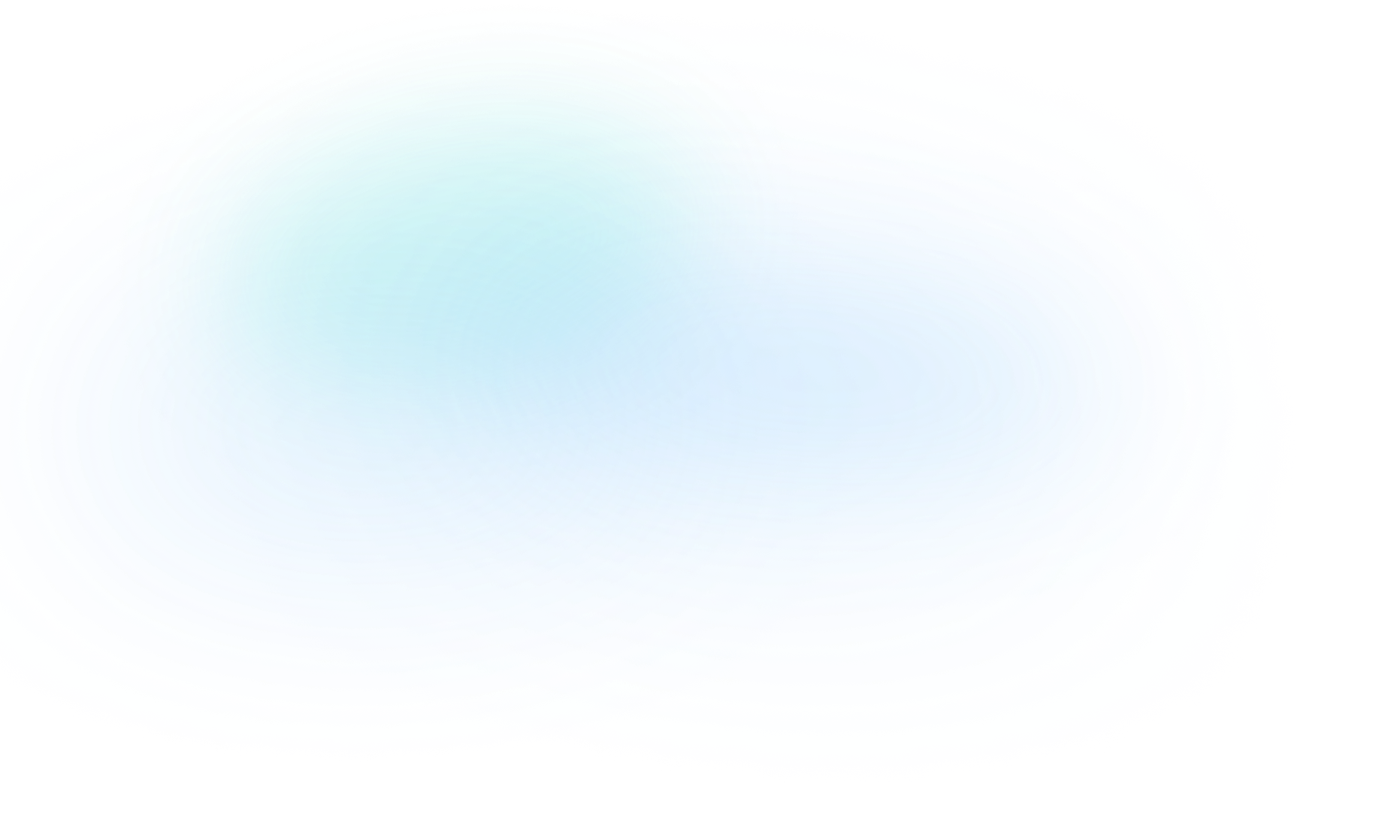
SCIENCE BEHIND THE MODEL
Quality of Hire Conquered
Crosschq is the only tool that provides complete Hiring Intelligence: from establishing a reliable Quality of Hire metric, to transformative, predictive insights that tie Quality of Hire to business outcomes.
GET A DEMO.png)
From Disparate Data to Actionable Insights
Evaluate the efficacy of your hiring strategies. Monitor and analyze data from initial candidate application through to their development as employees.

Key Machine Learning and Large Language Models Process & Summarize Data
Crosschq’s AI (Q) encompasses a variety of artificial intelligence methods used to solve diverse problems around Quality of Hire, serving 3 primary functions.
Crosschq’s AI (Q) encompasses a variety of artificial intelligence methods used to solve diverse problems around Quality of Hire, serving 3 primary functions.
Define the business outcomes that are correlative to employee outcomes
Correlate skills, competencies, experience, and more to positive outcomes
Determine how current hiring processes are correlated to positive outcomes
Four Inputs to a Quality of Hire Model
Dr Steve Hunt, Crosschq Scientist in Residence, has devised a rigorous model of four categorized inputs to calculating and correlating Quality of Hire. These moderators and influencers all feed into this perpetually operating model, allowing clients to leverage powerful insights from ongoing analysis and machine learning.
.png)
Machine Learning and Large Language Models
Process & Summarize Data
Machine Learning and Large Language Models
Process & Summarize Data
Crosschq’s AI encompasses a variety of methods to solve diverse problems around Quality of Hire, serving 3 primary functions:
- Define what post-hire outcomes are actually correlative to the employee outcomes you’re measuring
- Ascertain the correlation of candidate data such as skills, competencies, prior experience, education, and more, to positive outcomes
- Determine how your current hiring processes such as selection, interviewing, assessment, processes are correlated to positive outcomes
Explainable Algorithms & Validated Hiring Outcomes
Perpetually operating models leverage insights from ongoing analysis and adjust as new data becomes available.
Crosschq is vigilant to ensure fairness, transparency, and safety in our models. Supervised learning and continuous active monitoring, testing and training are in place to safeguard the system and check that it behaves as expected and bias is eradicated. Results can be trusted and verified.
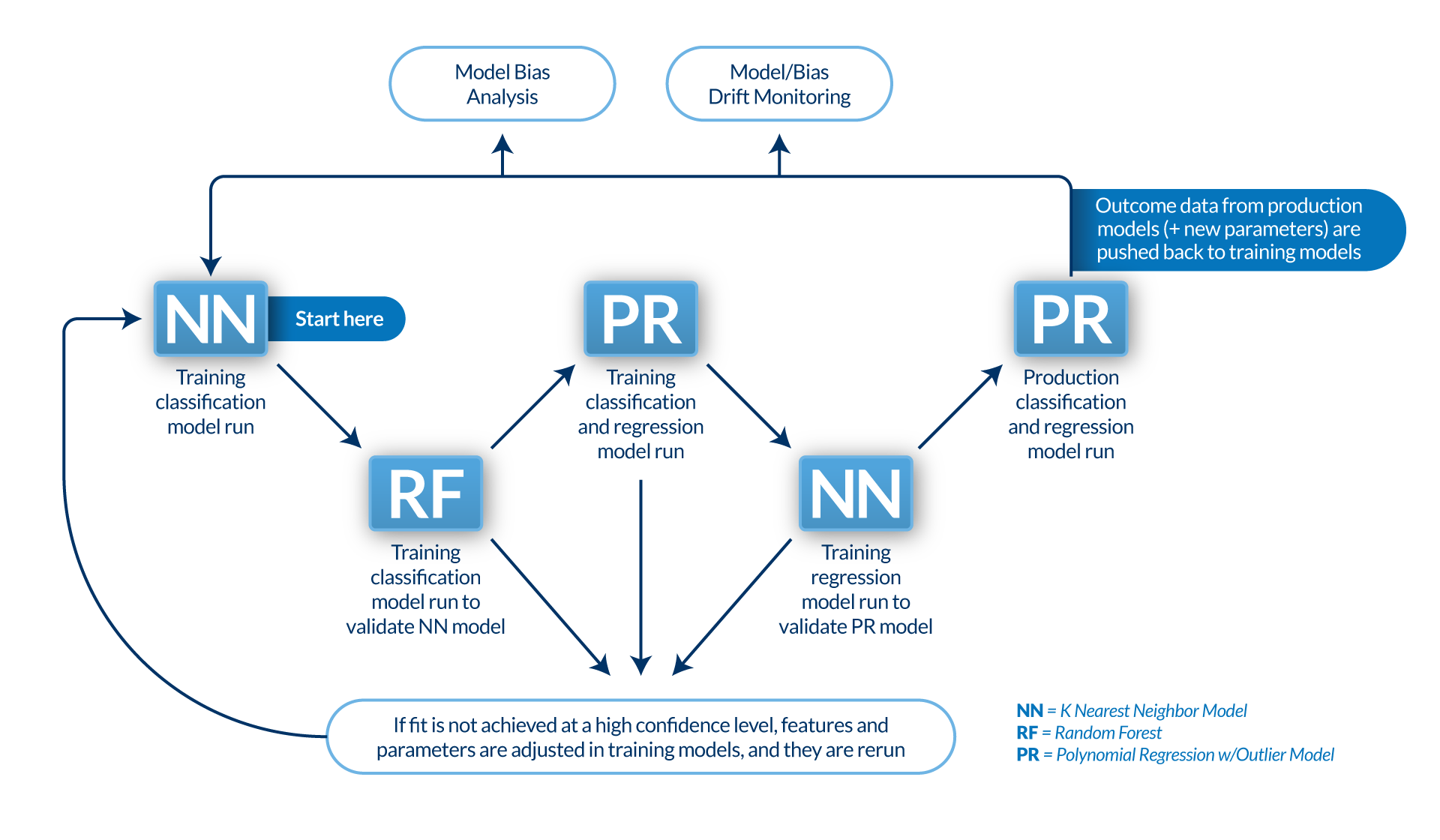
Customizable
Pre-Built Models
Crosschq provides four initial off-the-shelf models that are pre-built and validated for common organization situations including High Volume Hiring and Internal Mobility. These models are easily customizable based on the organization's specific goals, open role and desired outcomes.
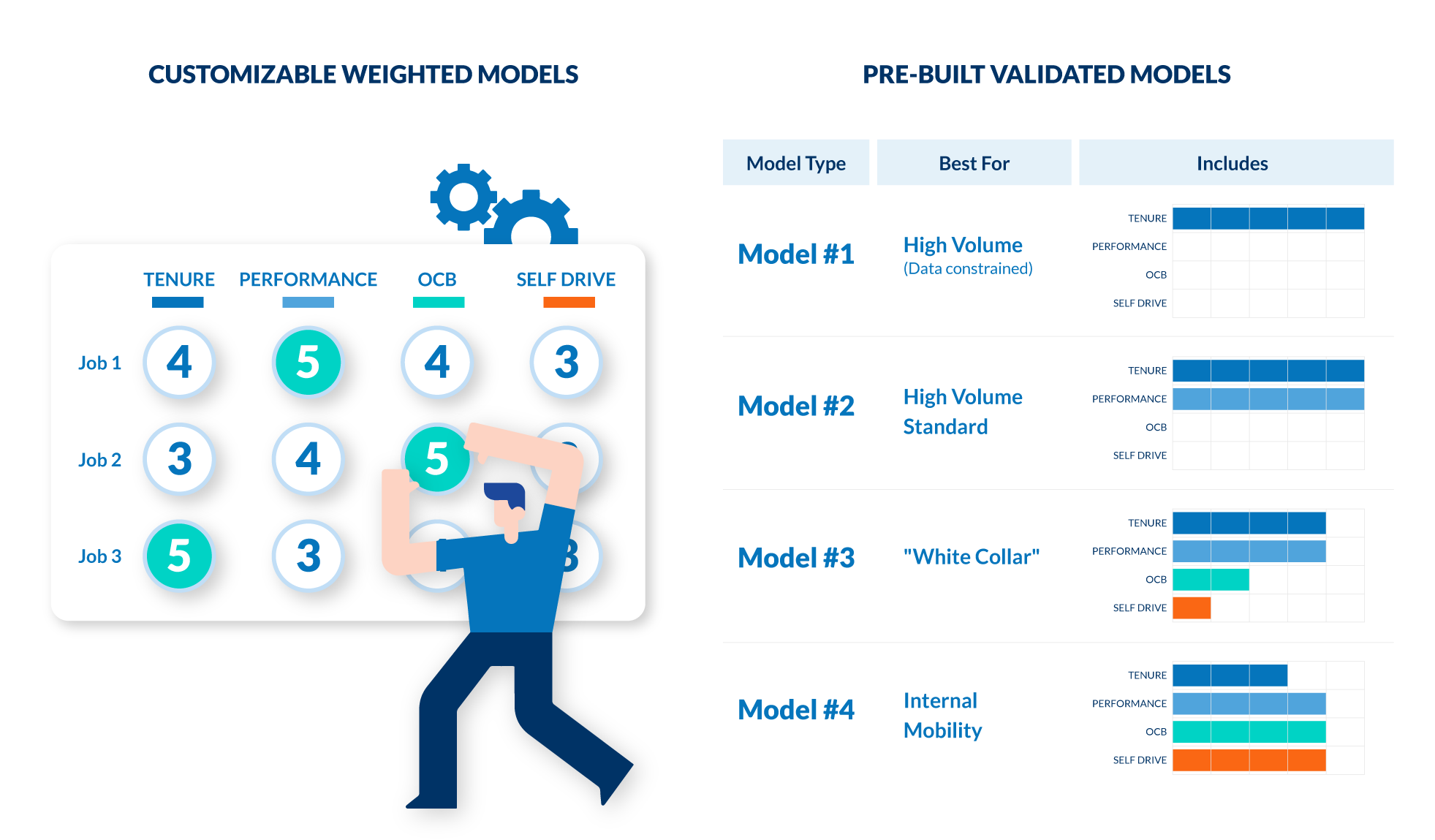
-
Post-Hire Outcomes
-
Candidate Data
-
Hiring Processes
.png)
Define the business outcomes that are correlative to employee outcomes your candidates

Correlate skills, competencies, experience, and more to positive outcomes

Determine how current hiring processes are correlated to positive outcomes
FAQ: Trust & Safety in AI
Ensuring fairness and minimizing bias in Crosschq's AI is paramount due to ethical, legal and practical considerations.
Ethically, Crosschq’s AI systems could wield significant influence over candidates and employees’ livelihoods, necessitating alignment with societal values of fairness, justice, and equality. Thus, compliance with regulations governing fairness, non-discrimination, and privacy is essential to Crosschq and our customers. Moreover, fostering trust and transparency among our users and stakeholders is contingent on the trust of our AI systems as fair, transparent, and accountable.
-
Crosschq is committed to fairness, accountability, and transparency in all aspects of our AI solutions.
Crosschq uses multiple fairness metrics to identify potential issues including statistical parity when measuring the difference in outcomes (e.g., candidate conversion rates, employee performance projections) between different demographic groups (e.g., gender, race, location) to assess whether outcomes are distributed equally across groups.
-
Crosschq constantly and consistently addresses issues such as demographic biases, algorithmic discrimination, and unfair outcomes.
-
Crosschq works to ensure training data includes diverse perspectives, demographics, and contexts to safeguard that the AI models are inclusive and equitable.
-
Crosschq has implemented feedback mechanisms that allow customers to report concerns or issues related to fairness and bias in our AI applications.
-
Multiple 3rd party experts auditing and validating models including Steve Hunt, PHD, former Chief Expert of Technology and Work at SAP and AI investment firm, Rocketship VC.
Take the
Guesswork
Out of Hiring
Schedule a demo now
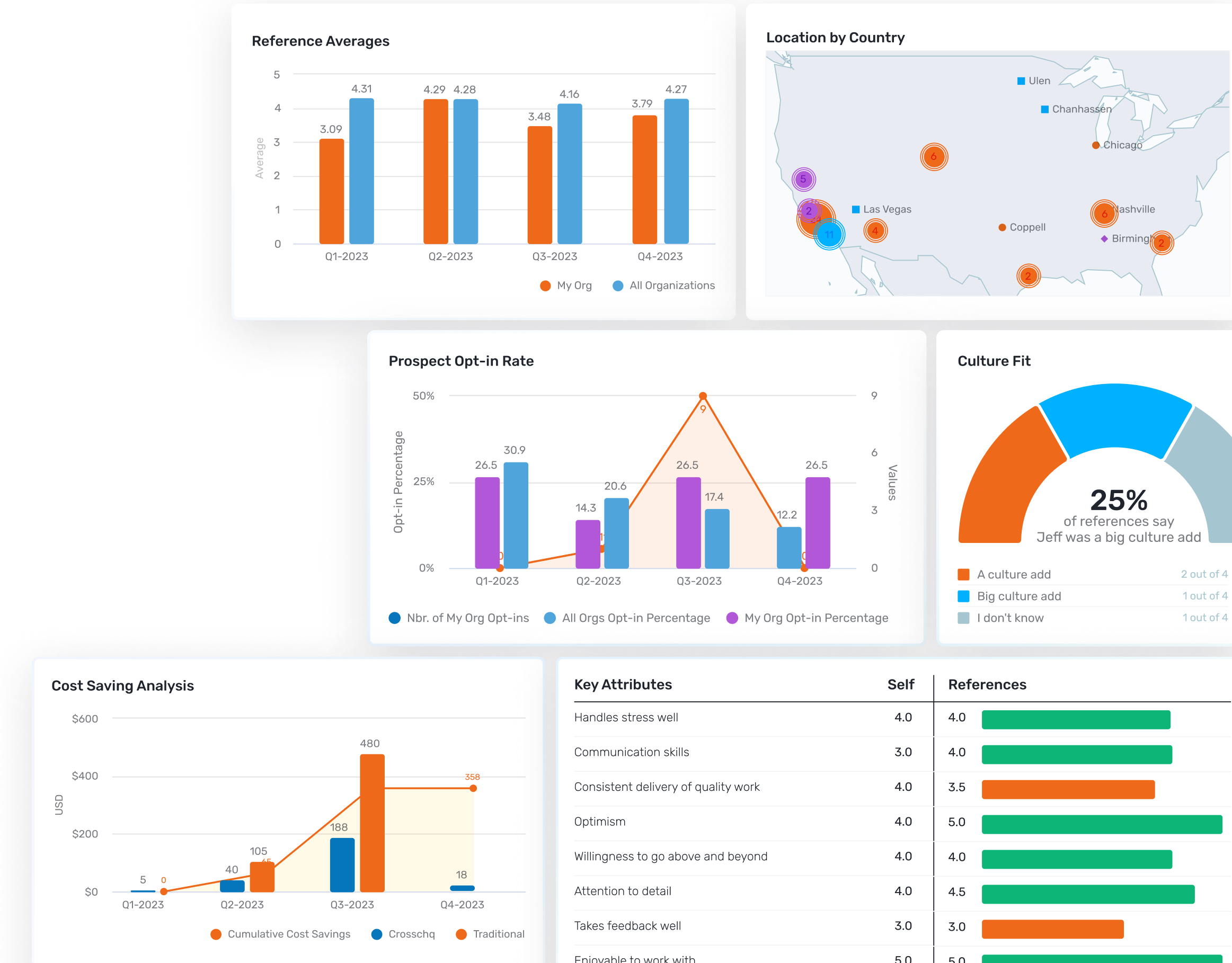
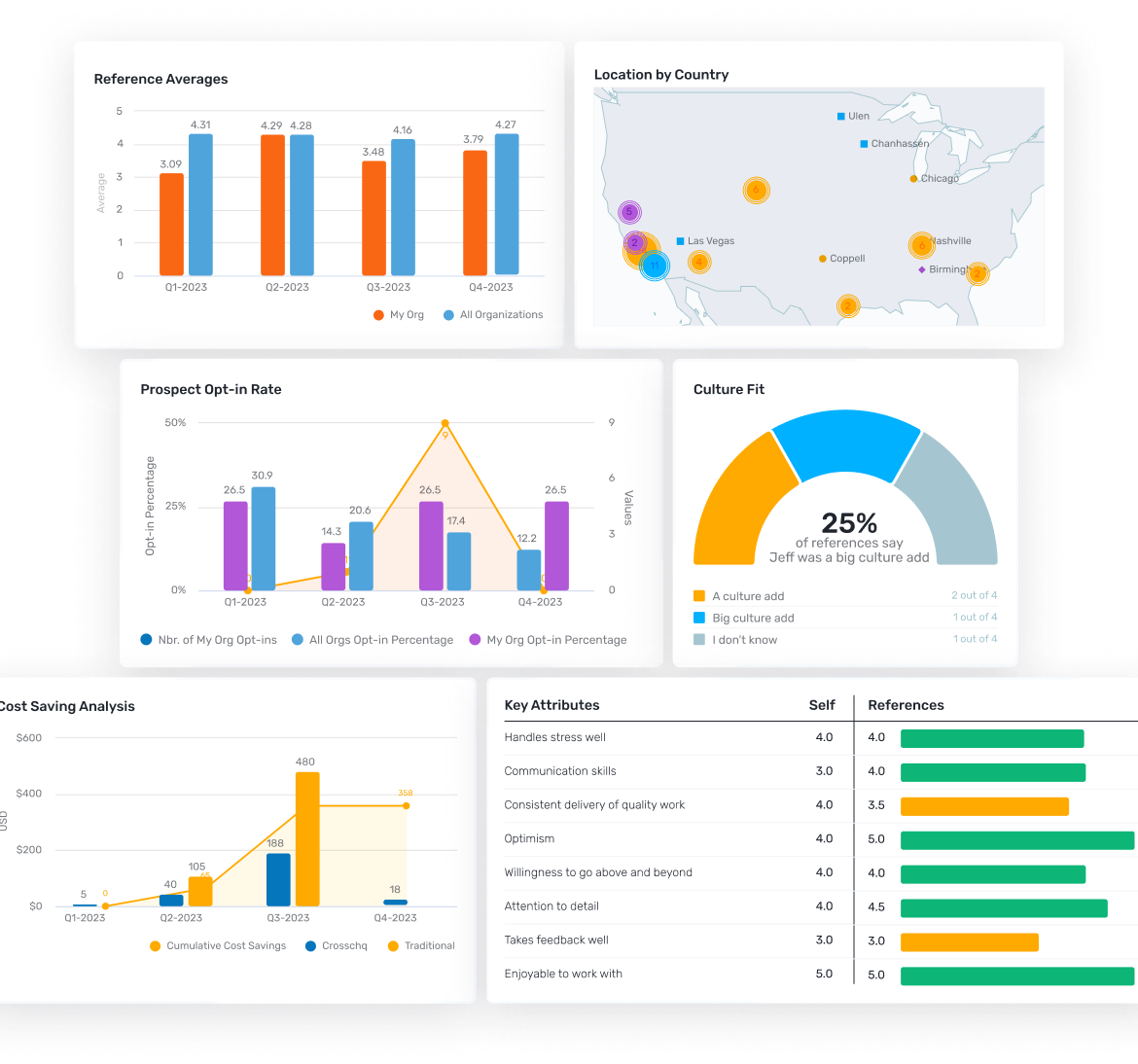
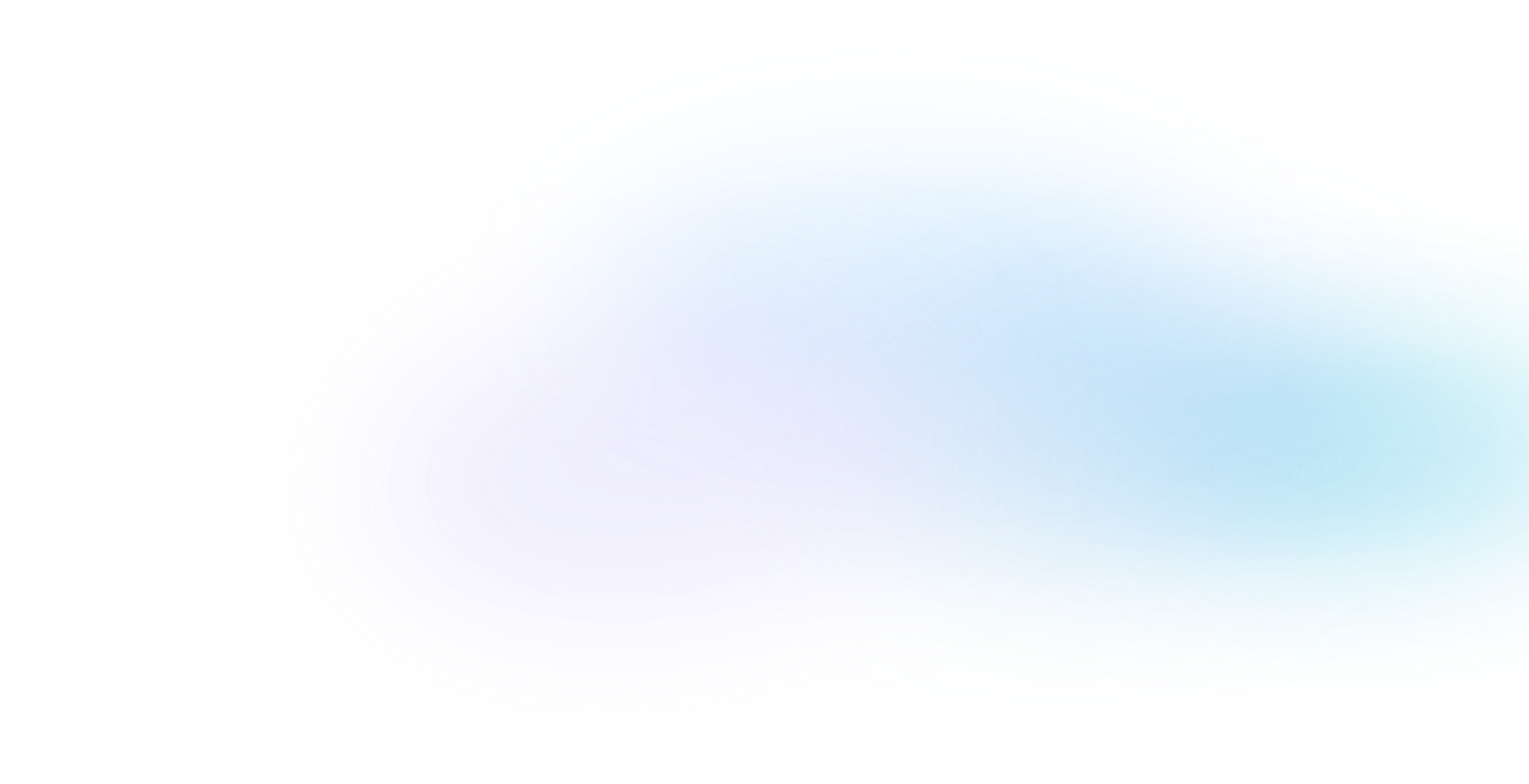